June 3, 2023: AI Safety
Good afternoon. Today we’ll take another crack at a topic that is hot at the moment: artificial intelligence safety. I have written on this subject several times already, most extensively in criticizing the idea of a six-month AI research pause.
A few weeks ago, Sam Altman, CEO of OpenAI, testified to the U.S. Senate in favor of regulations.
Altman suggested the US government might consider licensing and testing requirements for development and release of AI models. He proposed establishing a set of safety standards and a specific test models would have to pass before they can be deployed, as well as allowing independent auditors to examine the models before they are launched. He also argued existing frameworks like Section 230, which releases platforms from liability for the content its users post, would not be the right way to regulate the system.
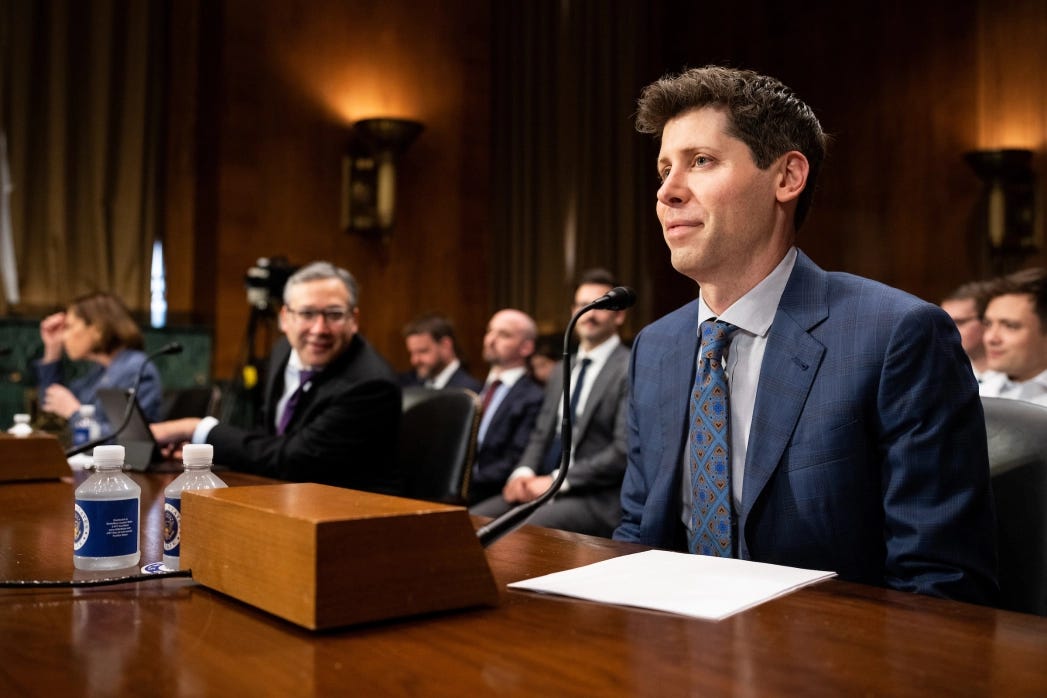
He appeared with Gary Marcus, a long-time critic of the deep learning technique on which ChatGPT, OpenAI’s flagship product, is based. All this calls to mind Bruce Yandle’s Bootleggers and Baptists thesis, which he put forward in 1983. Yandle’s argument is about how unlikely coalitions come come together to advocate for regulation. The title refers to how two groups—bootleggers and Baptists—have an interest in Sunday closing laws for bars and liquor stores. The interest is pecuniary for the former group and moral in the latter, but common interests are enough to put the two groups on the same side. Indeed, Yandle argues that the bootleggers and Baptists phenomenon is exactly what is going on now with AI regulation.
Altman’s ulterior motive should be obvious. The kinds of regulations he proposes, such as licensing for AI models, would create a regulatory moat. That is, they would create a situation where the cost of complying with regulation is so high that only large, established companies, such as OpenAI, will be able to do so. This is not a novel insight; regulatory moats, and the tendency for industry incumbents to lobby for them, have been well-known for a long time.
I would posit some principles for responsible AI regulation.
The “what” needs to be defined. The very term “artificial intelligence” is vague. It works to support a fluid research community, but precision is needed to write regulation. The term, by analogizing certain classes of algorithms to natural cognition, is a misleading anthropomorphism that contributes to public fear.
The “why” needs to be defined. As discussed in April, there are many distinct issues that are lumped under the banner of AI safety. The appropriate response to each of them is very different. When faced with a complex problem, it is best to decompose it into smaller pieces, but the banner of AI safety does the opposite. I’ve recently discussed some of the issues, such as technological unemployment, misinformation, autonomous weapons, and the alignment problem, and I plan on addressing more issues in the future.
The “how” needs to be defined. Vague calls of “we need regulation” are hard to take seriously if one does not know what that regulation would look like. Advocates of regulation in general, with AI regulation being no different, tend to be naive about the costs and the unintended consequences of what they propose. I would recommend Adam Thierer’s flexible approach as better thought out than most.
The AI safety label attracts people with general anti-technology views and people who see it as a platform for waging a culture war. Those who are trying to come up with responsible solutions need to disassociate themselves from malign elements, lest they find themselves irredeemably discredited.
Recently, the Center for AI Safety put out a statement,
Mitigating the risk of extinction from AI should be a global priority alongside other societal-scale risks such as pandemics and nuclear war.
Okay… That’s the full extent of the statement. Unlike the AI pause, which offered bad proposals, this offers no proposals at all. It’s the kind of milquetoast statement that few people are going to be against in the abstract, and judging from the list of signatories, I’m guessing that at least a few prominent names are there more for social reasons than reasons of conviction. It’s interesting that they chose pandemics and nuclear war as the prototypical risks to which they analogize. Other risks could have been chosen, which might significantly alter how the issue is conceived.
Finally, I am not going to let the AI safety topic pass without commenting on a major recent embarrassment for the movement. A few days ago, the press was full of sensational articles like this one about how, in a simulation conducted by the United States Air Force, an AI system was instructed to destroy enemy SAM (surface-to-air missile) sites, and when the commander ordered the system not to, the system killed the commander. When the system was retrained with a large penalty for killing its own commander, it destroyed the communication tower.
It turns out this never happened. After the story went viral, the Air Force clarified that the incident, based entirely on comments from Colonel Tucker Hamilton, was only a thought experiment. This article from TechCrunch, written before the denial, illustrates how the AI used in the alleged simulation was based on a reinforcement learning strategy that is already widely known among the research community to be flawed.
At a time when public panic about AI is high, fearmongering is deeply irresponsible and severely undermines any responsible effort to come up with safety solutions. It is also an indictment of modern journalism that so many news outlets passed along this story without asking basic questions, such as, you know, whether it actually happened. It is also a good reminder to, on any topic, apply common sense before sharing information.
Quick Hits
This week, Matt Yglesias wrote a piece on misinformation that would have been relevant to my post last week. He examines the prevalence of misinformation across the political spectrum. Ilya Somin commented and expanded on Matt’s piece a few days later. These pieces inadvertently raise another point on the subject. The label “misinformation” can be over-applied. I take it to refer to deliberate dishonestly or reckless disregard for the truth in presenting something, and so merely being wrong does not necessarily cross the line into misinformation. This definition requires inference about the motive of the source presenting information, and so it is easy to assume the worst.
Recep Tayyip Erdoğan recently won re-election in Turkey by a narrower margin than most were expecting. Daron Acemoglu, who has criticized what he characterizes as Erdoğan’s authoritarian rule, wrote this analysis after the runoff. Not discussed by Acemoglu is that hostility to Syrian refugees was a signature issue in challenger Kemal Kılıçdaroğlu’s campaign.
John Cochrane wrote this on work incentives for social programs; for SNAP (Supplemental Nutrition Assistance Program, or food stamps) and TANF (Temporary Assistance for Needy Families), they are part of the recently passed Fiscal Responsibility Act to raise the debt ceiling and avert an imminent default. Most social programs are means tested, meaning that if a person gains income through employment, they lose benefits from the programs, which Cochrane argues creates a disincentive to work. Here is a brief summary of the larger bill.
For Quillette, George Case wrote about how Noam Chomsky’s work provides an intellectual foundation for the kind of suspicion of mass media that has become a staple of both left-wing and right-wing populist movements.
[The state has] to control what people think. And the standard way to do this is to resort to what in more honest days used to be called propaganda. Manufacture of consent. Creation of necessary illusions. Various ways of either marginalizing the general public or reducing them to apathy in some fashion. (From Manufacturing Consent: Noam Chomsky and the Media)
It is a good illustration of how horseshoe theory is a better model for understanding politics than the traditional left/right spectrum.