Good morning. Note today’s date and be on the lookout for a “celebration” thereof.
The AI Pause
This week, several major researchers in artificial intelligences, including Stuart Russel, Yoshua Bengio, and Gary Marcus, and several other major influential figures, including Elon Musk, Steve Wozniak, Yuval Noah Harari, and Andrew Yang, signed an open letter calling for a pause “for at least 6 months” on training large language models. The letter invokes fear of what may happen with the development of AI systems, and it calls for the following:
These should at a minimum include: new and capable regulatory authorities dedicated to AI; oversight and tracking of highly capable AI systems and large pools of computational capability; provenance and watermarking systems to help distinguish real from synthetic and to track model leaks; a robust auditing and certification ecosystem; liability for AI-caused harm; robust public funding for technical AI safety research; and well-resourced institutions for coping with the dramatic economic and political disruptions (especially to democracy) that AI will cause.
Before we get into the letter itself, let’s look at some issues around AI safety in general, based mainly on references that the letter itself provides, though here’s a brief, easy-to-read intro from a few years ago.
The central risk that worries some people is “alignment”, the idea that a highly-capable AI system might pursue instrumental goals that are contrary to the intentions of the developer. (This is distinct from the problem that the developer’s goals themselves may be malicious.) Nick Bostrom’s paperclip maximizer has become a prototypical, humorous illustration of this issue.
In more recent work, wireheading refers to a situation where a reinforcement learning system may institute rewards that are distinct from those intended by the referent. It reminds me of this talk by Melanie Mitchell a few years back where a genetic algorithm was able to “succeed” with its training data by finding the address in memory where it is stored and deleting the data. Richard Ngo and coauthors discuss the problem in the context of deep learning, particularly relevant to GPT models and most contemporary work in artificial intelligence. Joseph Carlsmith estimates the odds of an existential catastrophe occurring by this mechanism by 2070 as at least 10%.
I find discussions about alignment-related risks to be highly speculative and unconvincing. Most discussion doesn’t demonstrate a very keen technical grasp. Of the things in the future that I worry about, AI misalignment is pretty far down the list. But I can’t rule out the risk entirely.
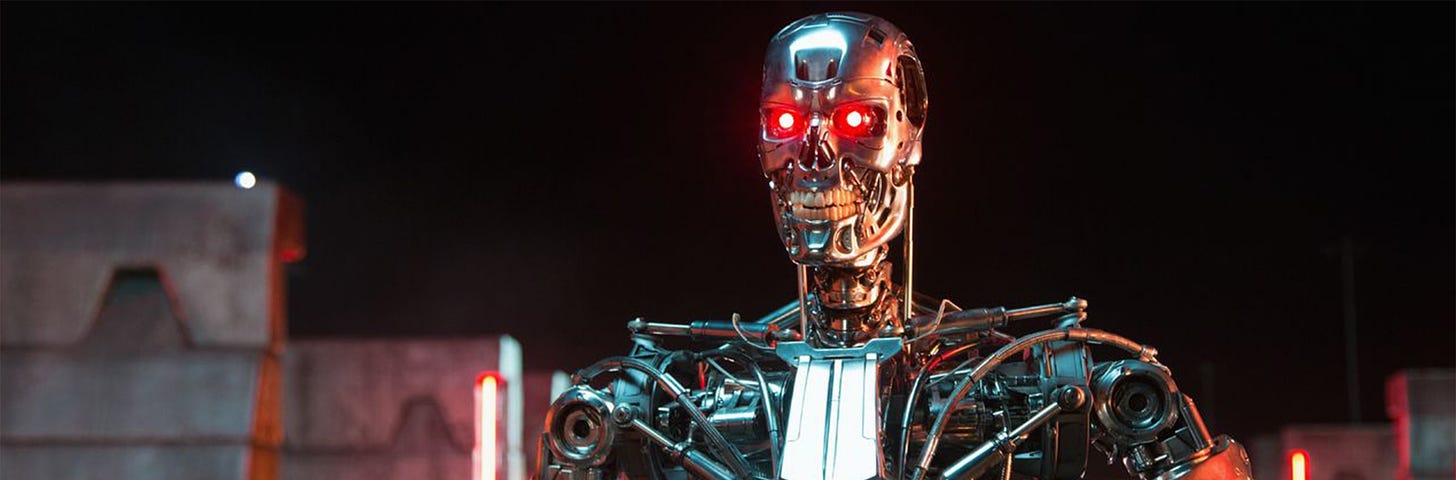
On other alleged existential risks from AI, people worry about the weaponization of these systems. I’ve discussed autonomous weapons twice before and why I think restricting their development would be ill-advised. See this paper by Hendrycks and Mazeika, especially pp. 13-14, for discussion of these risks. The paper is a valuable treatment of the topic, even if I dispute some of the specifics. This paper by Bucknall and Dori-Hacohen asserts that that AI could exacerbate conflict, could assist in the development of bioengineered pathogens, and could help conduct cyberattacks and foil cyber defenses through faulty training data. I don’t find their assertions convincing.
Moving on to risks that are not existential threats but potentially serious, the same paper by Bucknall and Dori-Hacohen argues that AI might shift geopolitical power to China, might shift political power from governments to corporations, will enable mass surveillance, and will pollute the information space with misinformation and deep fakes. There isn’t much discussion of the seriousness of the issues. Also, a reader who does not share the authors’ political orientation will notice this fact and find it hard to overlook. Weidinger et al. discuss many of the issues mentioned above, as well as concerns about bias, automation of jobs, and environmental impact. I’ll deal with another issue in the next topic.
Much more could be said, and might be said in the future, but to sum up, as a major general purpose technology, artificial intelligence poses a range of concerns. The central concern is of alignment, and around that is a constellation of other risks. Some of them are serious problems, some are not; some call for technical solutions in how AI is developed, and some call for other kinds of solutions.
Now we come to the letter. When it comes to the problems with the AI pause idea, I hardly know where to begin.
What is the most important aspect of any regulation? It should have a clear problem that it is trying to solve. The letter doesn’t make it clear what the problem is; it merely asserts that AI is dangerous and should be restricted.
What is the second most important element of any regulation? It should offer a clear solution. In the list of solutions quoted above, there are a few specifics, and some are hopeless vague. “[N]ew and capable regulatory authorities dedicated to AI”, to do what?
There is no consideration of what this will cost. James Pethokoukis of the American Enterprise Institute, quoting a Goldman Sachs report, states “Generative AI could eventually increase annual global GDP by 7 percent, equal to an almost $7 trillion increase in annual global GDP over a 10-year period.” And so the cost of a 6 month pause might be $350 billion. That’s a wild guess, but it’s better than no guess at all.
The Chinese government has identified AI as an important strategic area and is investing heavily. What’s the plan to convince the CCP to abide by the pause? And if they don’t, which they surely won’t, how does handing an advantage to the CCP help with AI safety?
Concerns about AI safety have been around for decades, and the community has still not put forward concrete solutions. How long it will it take to not only come up with solutions, but to draft the legislation and set up the regulatory apparatus to implement the solutions? A lot more than six months I’m sure.
How will the pause help come up with solutions? Couldn’t the Future of Life institute draft regulations with or without a pause? Why haven’t they done so already?
Regulatory capture is a thing.
The Shirky Principle is a thing too. Two of the proposed solutions are “robust public funding for technical AI safety research; and well-resourced institutions for coping with the dramatic economic and political disruptions (especially to democracy) that AI will cause.” No doubt by “well-resource institutions” the Future of Life Institute is thinking about itself. They will have a clear incentive to not solve supposed risks from AI and to manufacture new risks to keep the money flowing.
The AI safety movement is a perfect vehicle for interests that oppose artificial intelligence for ideological or pecuniary reasons. Judging from the list of signatories, this has already happened. Thus AI safety is transforming into an anti-AI movement.
The Pause is not even close to being a workable idea. Whoever drafted this letter shows profound ignorance of basic issues in politics, geopolitics, and economics. This is what happens when an epistemically closed movement tries to draft policy. And the pretense is downright laughable. Consider, “Unfortunately, this level of planning and management is not happening”, and
Should we let machines flood our information channels with propaganda and untruth? Should we automate away all the jobs, including the fulfilling ones? Should we develop nonhuman minds that might eventually outnumber, outsmart, obsolete and replace us? Should we risk loss of control of our civilization?
Who is the “we” here? Is it the royal we? Or is it humanity in the collective, on whose behalf the Future of Life Institute, despite their ignorance of basic societal issues, claims the authority to speak? Words like “planning” and “management” and “control” invoke a technocratic vision of how society should operate.
Also this week, Eliezer Yudkowsky wrote a vile editorial in Time calling for all AI work to be shut down permanently (bold mine).
Shut down all the large GPU clusters (the large computer farms where the most powerful AIs are refined). Shut down all the large training runs. Put a ceiling on how much computing power anyone is allowed to use in training an AI system, and move it downward over the coming years to compensate for more efficient training algorithms. No exceptions for governments and militaries. Make immediate multinational agreements to prevent the prohibited activities from moving elsewhere. Track all GPUs sold. If intelligence says that a country outside the agreement is building a GPU cluster, be less scared of a shooting conflict between nations than of the moratorium being violated; be willing to destroy a rogue datacenter by airstrike.
I mention this to illustrate just how detached from reality some elements of the AI safety movement have gotten. Conducting airstrikes against data centers in other countries is supposed to enhance safety? Does he really not realize how insane this sounds to most people? For those of us who remember the 1990s, this kind of thinking is familiar. Shame on Time for publishing this.
Two more responses: from Tyler Cowen at Marginal Revolution and from Ronald Bailey at Reason.
Insofar as there are serious risks from AI, neither the Future of Life Institute nor anyone with significant involvement with this letter has shown the judgment necessary to address these risks.
Hunter-Gatherer Leisure
How much leisure time did hunter-gatherer societies typically have? And what does ChatGPT have to say about it?
Since the 1960s, several anthropology papers and popular books, such as Jared Diamond’s Guns, Germs, and Steel and Yuval Noah Harari’s Sapiens, have endorsed the idea that hunter-gatherers typically worked around 20 hours per week. As far as I can tell, the idea comes from Marshall Stahlens, who coined the term “original affluent society”, a play on John Kenneth Galbraith’s The Affluent Society, as presented in the Man the Hunter symposium, and synthesized his ideas in his 1972 Stone Age Economics. Another major source for this idea is Robert Lee’s study of the !Kung San in northern Botswana, who Lee argues worked around 2.4 hours per day on average. The idea is also presented by Peter Just. Antecedents go back at least as far as Jean-Jacques Rousseau’s noble savage.
Rachel Lauden documents that the !Kung San spend 3.5-4.5 hours per day processing the food, making and repairing tools, butchery, cooking, and fuel collection. This includes 8 hours a day cracking mongongo nuts, which doesn’t sound like a lot of fun. None of this was included in Lee’s estimate.
In 2000, David Kaplan reviewed some of the same points about how hunter-gatherer leisure is greatly exaggerated, and more broadly critiqued Stahlens’ “original affluent society” notion. Kaplan also points out that !Kung San statures tend to be small, indicating nutritional deficiencies. They were particularly lacking in animal fat.
Bhui, Chudek, and Henrich find specifically “Shifting to fully commercial labor is associated with an increase in men’s work from around 45 h per week to 55 h, on average; women’s work remains at nearly 55 h per week across the spectrum” in data collected across eight small-scale groups around the world in the late 1970s and 1980s.
In other recent work, Dyble et al. look at Agta hunter-gatherers in the Philippines. “We find that individuals in camps engaging more in non-foraging work spend more time involved in out-of-camp work and have substantially less leisure time.” The paper points out,
Also, the Agta are able to increase the returns on foraging by trading with their non-Agta neighbours; they trade or sell approximately a quarter of all foraged food (and half of all foraged fish and meat) for rice and other agricultural products. This kind of protein-for-carbohydrate exchange is common between contemporary foragers and their farming neighbours and may also reduce reliance on wild carbohydrates. Finally, the livelihood of the Agta is influenced not only by their interactions with non-foraging neighbours but also by national policies relating to the status of indigenous people, land rights, and the environment.
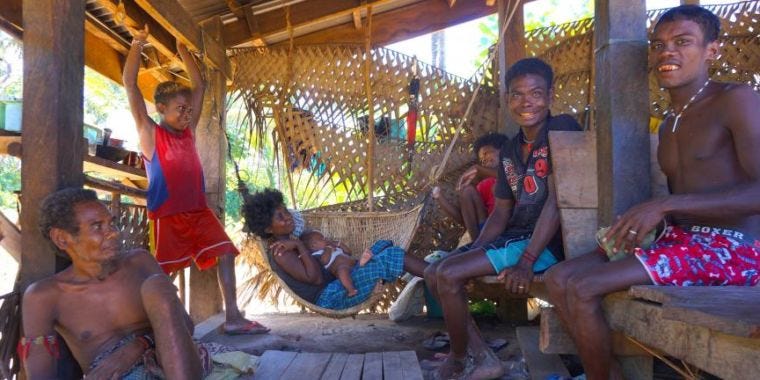
The obvious problem with all this is discussed by Robert Kelly: we should be very careful about extrapolating from contemporary hunter-gatherer societies to Paleolithic societies. I discussed this problem earlier in the context of large-scale cooperation in Pleistocene societies.
Working hours are only one piece in the “original affluent society” thesis of Stahlens. He argues that, despite being poor by contemporary standards, hunter-gatherers felt that their needs were met and by this definition they were “affluent”. On the subject, David Kaplan says,
One gets the impression that for many—perhaps most—anthropologists, the vision of hunter-gatherer affluence contains some profound insight into the human condition, and they therefore very much want that vision to be true.
After I had mostly done the research to write the above, a friend suggested that I ask what ChatGPT thinks of this, and so I did. I found the results to be illuminating and disturbing. In response to my first question, “How many hours did a typical person in a hunter-gatherer society work?”, ChatGPT gave what I think is now the conventional answer on the topic.
The number of hours worked by individuals in hunter-gatherer societies varied depending on a variety of factors, such as the environment, availability of resources, and cultural practices. However, anthropological research suggests that on average, members of hunter-gatherer societies worked between 20 to 30 hours per week to meet their basic needs for food, shelter, and clothing.
…
I then asked “What is the most compelling anthropological research on the topic?” and got back (bold added by me),
There have been numerous anthropological studies on the work hours of hunter-gatherer societies, and different researchers have presented various perspectives on this topic. However, some of the most compelling research has come from studies of contemporary hunter-gatherer societies that still exist today.
One well-known study is that of the !Kung people in the Kalahari Desert of Southern Africa. Anthropologist Richard Lee conducted extensive fieldwork among the !Kung in the 1960s and 1970s and found that they typically worked between two and a half to four hours per day on average to meet their basic needs. This work included activities such as hunting, gathering, and preparing food, as well as making tools and tending to their children.
Other studies of contemporary hunter-gatherer societies have reported similar findings. For example, anthropologist Marshall Sahlins conducted research among the Ju/'hoansi people of Botswana and Namibia in the 1960s and found that they worked an average of 20 hours per week. Anthropologist Brian Hayden has also conducted research among the Nunamiut people of Alaska and found that they worked an average of 27 hours per week.
These studies provide strong evidence that, contrary to popular belief, hunter-gatherers did not spend all of their time working to meet their basic needs. Instead, they had ample time for leisure activities, socializing, and cultural practices. This challenges the common assumption that early humans led lives that were "nasty, brutish, and short," and suggests that our ancestors had more leisure time than many people think.
Two of these I confirmed above. I know Brian Hayden worked with the Nunamiut people, but I am not aware of the specific reference. I bolded the “contrary to popular belief” phrase, because academics still present the hunter-gatherer leisure idea as though it was a novel insight, but this appears to be the popular belief by now.
We’re not getting off the hook that easily. I asked, “David Kaplan argues that Richard Lee greatly underestimates the working time of the !Kung because he fails to account for food processing time. What is your view on this?” and got back,
David Kaplan's critique of Richard Lee's estimates of the work hours of the !Kung people is certainly an important contribution to the debate on this topic. Kaplan argues that Lee underestimated the time spent on food processing, which includes activities such as grinding, pounding, and cooking food, and that this could significantly increase the total amount of time spent on work.
It is important to note that Kaplan's critique is not based on new fieldwork among the !Kung people but rather on a re-analysis of Lee's original data. In his response to Kaplan's critique, Lee has acknowledged that he did not include time spent on food processing in his initial estimates and that this could have added an additional two to three hours per day to the total work hours of the !Kung.
However, it is also important to note that the debate over the work hours of hunter-gatherer societies is not solely about getting an accurate number of hours worked. Instead, it is a broader discussion about the nature of work and leisure in these societies and what we can learn from them about human history and culture.
Regardless of the exact number of hours worked by the !Kung or other hunter-gatherer societies, it is clear that these societies had a very different relationship with work and leisure than modern industrial societies. They did not have a separation between work and leisure time, as many of their activities were both productive and enjoyable. Additionally, they had a much more egalitarian and communal approach to work, with individuals working together to meet their collective needs rather than pursuing individual goals.
Science done well means painstakingly gathering evidence, such as the on the working patterns of hunter-gatherer societies, and drawing conclusions from them. Science done badly means starting with a narrative and then gathering evidence to support it. To promulgate a narrative and treat the empirical evidence as irrelevant is not science at all. This is exactly the point Kaplan makes.
And ChatGPT says, “it is clear that these societies had a very different relationship with work and leisure than modern industrial societies”. Well no, it’s not clear. If it is difficult to discern working patterns of modern hunter-gatherers and extrapolate them to the Paleolithic, then it is virtually impossible with something intangible such as “relationship with work”.
I won’t relay ChatGPT’s reaction to Kelly’s work in the interest of space, but I found that unsatisfying as well.
Here’s what worries me. ChatGPT is very good at repeating the conventional wisdom on a topic. It is not good at evaluating claims critically and challenging conventional wisdom when it should. Large language models have allegedly shown racial and gender bias and bias against conservative political viewpoints. These biases are relatively easy to quantify and rectify, as there are large constituencies to do so. I am more worried about the biases for which there is not a well-defined constituency. In these cases, bad information can get laundered through an AI system, which gives it more gravitas and increases the difficulty of detecting it.