Quite a few new readers have joined in the last couple weeks, so welcome. My main focus in this blog now is to support my Scaling in Human Societies project, which is generously supported by OpenPhilanthropy. Over the almost five years I have been writing, my main focus has been on energy, environmentalism, and urban design, though I like to venture into other topics sometimes, such as a post a couple weeks ago on Operation Cyclone. I try to produce one post every week, though last month’s schedule was disrupted by an illness that is now hopefully passed.
This week’s post is very much part of the Scaling project. Last week, I considered some theoretical aspects of why larger cities tend to be more prosperous, on a per-capita basis, than smaller cities. This week, we’ll look at some empirical results and what this implies about how we think about cities.
The 7/6 Rule
The best place to start is with Luís Bettencourt’s The Origins of Scaling in Cities (2013). Bettencourt is a physicist and complex systems researcher, a professor at the University of Chicago, and an external professor at the Santa Fe Institute. The physics perspective very much shines through in his paper.
The main accomplishment of Bettencourt (2013) is the derivation of theoretical predictions of how various quantities related to a city scale with population. These quantities include that socioeconomic rates—including economic output per capita—of a city should grow with the 7/6 power of the population of a city. Equivalently, per-capita output should grow with the 1/6 power. Also equivalently, if the population of a city doubles, then per-capita output, as well as wages, should increase by about 12%. Last week, I discussed some of the precise mechanisms by which this happens, and we know intuitively that large cities provide better jobs and more opportunities, which is why they exist in spite of their drawbacks.
Bettencourt’s model is based on four assumptions.
Mixing population. A resident at any point in the city should be able to commute daily to any other point in the city. As I discussed a few weeks ago, the city size is defined very roughly by Marchetti’s Constant (Marchetti (1994)), or a 30 minute commute radius around a central business district.
Incremental network growth. Because cities grow incrementally and naturally, infrastructure networks (roads, water, electric, etc.) are decentralized. A consequence of this, in light of the first assumption, is that travel paths are generally not straight lines, nor should residents at the city fringe expect highway speed travel to the city center.
Bounded human effort. One objection to urban scaling principles, that I hope to address more fully later, is that large cities are mentally taxing. Bettencourt avoids this problem in his modeling; how well it is avoided in real life is unclear to me.
Socioeconomic outputs (e.g. GDP) are proportional to social interactions. This captures the intuition that a city is not merely a collection of people who live near each other, but rather wealth is created through the interactions between them. This point is emphasized by Jacobs (1969).
The fourth assumption bothers me the most. My intuition is that there should be a declining marginal value to social interactions. In other words, if a city grows in such a way that fosters 25% more (potential) social interactions, then GDP should increase, but by less than 25%. If this is correct, then output should not scale quite as strongly as the paper argues.
The mathematics of Bettencourt’s work are important, but I haven’t figured a good way to present them in a layperson-friendly manner, and so I won’t attempt to do so. Scaling laws are the consequences of the mathematization of these assumptions. Bettencourt derives a 7/6 power law (1/6 on a per-capita basis) for socioeconomic quantities such as GDP as mentioned above. Further consequences are that area scales with the 2/3 power of population, and that infrastructure network lengths, such as the number of lane-miles of road, scale with the 5/6 power of population.
Another basis for doubt about Bettencourt’s calculations is that they treat cities as closed systems. They do not account for interactions between cities, but clearly an individual’s social network includes relationships and interactions with people in other cities or even on other continents. Taking intercity interactions into account should offer a comparative advantage to smaller cities and further dampen the actual scaling relationship.
Actual Scaling Results
OK, enough theory. Let’s look at some actual numbers, and we’ll start again with Bettencourt (2013). He examines gross metropolitan product in 2006 for 363 metropolitan areas in the United States and performs a regression against population size. He finds that the slope of the line on a log-log plot is 1.126 ± 0.023. Note that the theoretical value is 7/6 = 1.16666…, which is not within the margin of error. This makes me wonder if the doubts expressed above are valid. But obviously there is a real scaling relationship here. The R² value is 0.96, which is very high, suggesting that population is a good predictor of output. In statistics, R² is a common measure of the goodness of fit of a prediction. An R² value of 0 means that the model has no predictive power whatsoever, while an R² value of 1 means that the prediction is perfect.
Bettencourt (2013) also examines the number of lane-miles of 415 urban areas in the United States. Again, he performs a regression on the log-log plot of lane miles and population, and he finds a slope of 0.849 ± 0.038. This time, the theoretical value of 5/6 = 0.83333… is well within the margin of error, though the R² value is a less impressive 0.65.
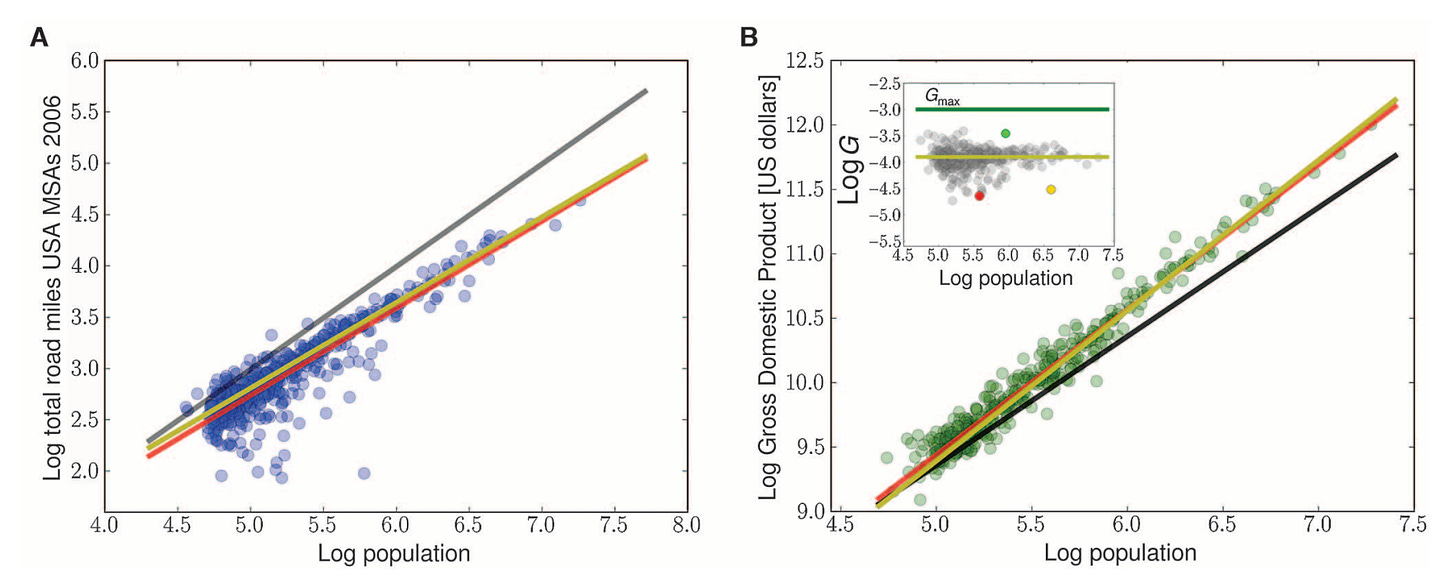
Lobo et al. (2013) (Luís Bettencourt is one of the authors) perform a similar analysis, this time bringing into consideration smaller cities, or micropolitan statistical areas as defined by the U.S. Census Bureau. They find that the slope of the output/population regression line is 1.146, close to the theoretical prediction, with an impressive R²=0.97. There is no obvious pattern to the outliers. The most extreme is Los Alamos, New Mexico, which is a small city that receives a large amount of federal research investment with the Los Alamos National Laboratory, going back to the Manhattan Project.
This would be a good time to comment on another objection to scaling laws. As stated in Lobo et al. (2013),
To be clear, we do not claim that there is a causal relation between urban scaling and urban productivity; scaling reveals a systematic relationship between urban population size and productivity, which itself is a manifestation of a more general relationship between population size and productivity.
If larger cities are generally more productive than smaller cities, how do we know that it is because they are larger? Causation could plausibly go in the opposite direction. Some cities are more productive because of geographic advantages, better governance, or other idiosyncratic factors that are unrelated to their population, and improved productivity attracts residents, thus creating the relationship between productivity and size. Based on the theoretical model of Bettencourt (2013) and many empirical results, I am inclined to believe that size actually does cause productivity to a large extent, but the objection is worth putting out there. Melo et al. (2016) is among the papers that address this issue.
Speaking of Melo et al. (2016), they approach the question of agglomeration on a level below that of a full metropolitan area. Considering data cross the largest 50 metros in the United States, they estimate the elasticity of wages (a decent proxy for output) with employment density—the number of jobs available in a given area—and employment access—the number of jobs available in a given travel time. They find that the elasticities are, respectively, 0.072 and 0.096. These numbers capture our observation that jobs opportunities are generally better in the center of a city than at the fringe, and they also capture the predictions of the standard urban model. Yet the elasticities are lower than those reported in the above papers, perhaps because, by looking at a level smaller than a full metro, they capture a smaller portion of a person’s social network.
Another finding of Melo et al. (2016) is that agglomeration economies attenuate with travel distance, with most of the benefit gone after 20 minutes of driving, though some benefit persisting up to 60 minutes. Though not made directly in the paper, one point I take away is the importance of having an efficient road network. Simply having a large number of people living in a small area does not make for a well-functioning city, as defined by agglomeration economy, if transportation around the area is hampered by gridlock and circuitous road networks.
The last paper we’ll consider today is Schläpfer et al. (2014). This paper does not consider economic output directly, but rather it examines social interaction, for which mobile phone connectivity is taken to be a good proxy. They find that in Portugal, the 92-day call volume scales with the 1.10 (1.08-1.11) power of city size, whereas in the United Kingdom, the 31-day call volume scales with the 1.10 (1.07-1.14) power of city size. There are various other metrics related to the call data set, all of which show similar scaling properties. One thing especially interesting about the paper is that they find that, for two of a given person’s contacts, the probability that they are themselves connected is independent of city size, which challenges the popular notion that larger cities are more atomizing than small towns.
How to Think About City Size
There are a ton of papers on this subject, and I plan to discuss more of them later on. But for now, I would like to conclude with some thoughts about city size from a policy perspective.
There are numerous results about how desirable properties (e.g. output, wages, innovation, social amenities) grow with city size, and how undesirable properties (e.g. traffic congestion, crime, infectious disease, pollution) also grow with city size. Over the course of the Scaling project, I hope to discuss all of them. City size is determined by an equilibrium between desirable and undesirable properties. The benefits of cities are why we don’t live isolated lives like Robinson Crusoe, and the drawbacks of cities are why we don’t live in an 8 billion-person ecumenopolis.
One of the less appreciated achievements of the industrial revolution is how, by taming the drawbacks of size, we can capture the benefits of large cities. Noncombustible building materials (e.g. concrete and glass) and professional fire departments have mostly ended the great fires that devastated preindustrial cities. Professional police departments have tamed the rampant crime of earlier eras. Public health has lessened the disease epidemics of earlier eras, though the COVID-19 pandemic reminds us that the threat still exists. Modern pollution controls have likewise made density healthier and more pleasant. And transportation technology, including the unfairly maligned personal automobile, have allowed larger numbers of people to live together in a cohesive economic unit while allowing more personal space.
Good urbanism then, in my view, is about further managing the downsides. For this reason, I am particularly worried to see how many city leaders, and national leaders insofar as they have responsibility, show an unwillingness or inability to deal with chronic issues such as crime, public health, traffic congestion, and housing costs. If the downsides are managed well, then the upsides will follow naturally.
My biggest concern, though, is whether the prosperity that urban success generates also produces lifestyles and political ideologies that are ultimately not conducive to future success. Questions of sustainability are very much a part of discussion around urban design, and I share those concerns, even if I have a somewhat different take on the subject. But this is another topic for another day.
Quick Hits
At long last, I have prepared a draft of the learning curve paper that I was working on for much of the year. I was planning on finishing and sharing this right after the Markets & Society conference in October, but a combination of the start of work on the Scaling project and illnesses greatly delayed this. I have not submitted this paper to a journal as I had originally intended, and though I think the amount of effort that has gone into the paper merits a better treatment than being placed on GitHub, I am not sure what to do with it yet.
The growth of solar power this century has been impressive, but we can also compare actual performance with forecasts from the last decade. Farmer and Lafond (2016) stated that
A simple trend extrapolation of the growth of solar energy (PV and solar thermal) suggests that it could represent 20% of the energy consumption by 2027.
According to the Energy Institute Statistical Review of World Energy, solar generation in 2023 was just under 2.5% of primary energy consumption. That number won’t plausibly increase eight-fold in four years. Hanson, Partain, and Hanson (2015) projected that solar generation might reach 100% of electrical generation in 2015 by 2028; according to the Statistical Review, it was at 6.8% in 2023. A subtext of these wild forecasts, which were based on naive extrapolations of cost trends of the time, was that clean energy deployment was a solved problem, and that we need not worry about such things as permitting reform, nuclear power, carbon capture, or carbon pricing. They have ultimately done severe damage to the cause of climate change mitigation.
Nascimento, Kuramochi, and Höhne (2022) found that among G20 nations, 2030 greenhouse gas emissions were projected in 2021 to be 15% lower than projections for 2030 that were made in 2015. Of that reduction, about a quarter can be attributed to unforeseen effects of COVID-19. The authors attribute the rest of the drop to climate policies that have been adopted since 2015 and revised expectations about technology development and economic growth. It is not at all clear to me that the drop can be attributed to Paris commitments specifically, but the paper is one of a few to attempt to evaluate the effect that the agreement has had on the emissions trajectory. It will not surprise readers to hear that the authors assess that the new emissions projections are also far above what is necessary to meet the 1.5 °C global warming goal in the agreement.
Several big names in biotechnology have published an editorial in Science Policy Forum calling for a moratorium on efforts to develop mirror life. What is that? Mirror life employs nucleotides and amino acids with the opposite chirality of ordinary life. Although it is unknown if this would actually happen, the authors fear that mirror bacteria could cause infections in plants and animals, including humans, for which immune systems and drugs would be ineffective in treating, causing devastating damage. Names on the editorial include Craig Venter, who was a leader in sequencing the human genome, and George Church, a codeveloper of CRISPR-Cas9 and founder of many biotechnology companies. The letter is reminiscent of last year’s AI pause letter, about which I wrote critically, although in this case, I don’t understand the issues well enough to render an opinion. Jason Crawford has written a bit more about the subject.
There is reason for trepidation for the future of Syria, but this video captures jubilation on the streets of Damascus now that Bashar al-Assad has been forced out of power. The U.S. State Department documents horrific human rights abuses by the Assad regime, supported by Russian and Iranian allies. Hayat Tahrir al-Sham, the leading rebel group in this month’s blitzkrieg against the regime and the presumptive leader of the new government, fares badly as well, as do Turkish-backed groups in the north.
Any sort of political violence is detestable, but there is something especially revolting about violence directed at the health care system, as we saw recently with the murder of UnitedHealthcare CEO Brian Thompson. The assassin was evidently inspired by Ted Kaczynski (the Unabomber) and motivated by animus toward UHC as a company and the health insurance industry as a whole. The episode is reminiscent of “propaganda of the deed”, violence against high-profile individuals which Fleming (1981) identifies as central to turn-of-the-20th-century anarchism, an international terrorist movement. The fact that so many individuals have praised the assassination is even more disturbing, and it is another step down the dark road to a future where violence is seen as an acceptable means of political activism.
If you haven't read them, The Plague Cycle and Order Without Design are excellent connections to your work here on several points.
Kenny's Plague Cycle is mainly about overcoming the public health issues with density. It's an argument for and defense of globalization in the face of disease.
Order Without Design has a short discussion of city size that you've made me think is too optimistic. It has been years since I read the book, but my memory was a claim to one-to-one scaling of city population and output. However, Bertaud's conclusion is that planners should be much more thoughtful in how they think about city design. Again, my memory is fuzzy, but he advocated for a focus on quality of life measures instead of minimum lot sizes etc. That seems in line with your points about crime and costs of major cities.